Bayes Theorem Calculator
If you’re feeling a bit lost, read this introduction to Bayes Theorem, which shows when and where to use the Math.
Comparing Two Hypotheses
You’ve seen your colleague, Alice, be late 3 days, and on time 4 days the past week.
Hypothesis: They’re almost always late, i.e. they’re late 95% of the time.
Alternate Hypothesis: They’re almost never late, i.e they’re late about 5% of the time.
\[ Posterior \hspace{2mm} Odds = Prior \hspace{2mm} Odds * Likelihood \hspace{2mm} Odds\]
Main Hypothesis success rate | % |
Alternative hypothesis success rate | % |
Number of Successes | |
Number of Failures | |
Total | |
Likelihood Ratio (rounded) |
Everyone has their own prior odds. I used 1:1 for the being late case. But, you can play around with these too.
Prior Odds | : |
Posterior Odds |
In the being late example, this gives us that our current hypothesis is \(\frac{1}{19}\) times likely as the other one.
Comparing Two Hypotheses - known likelihoods
Prior Odds | : |
Likelihood Odds | : |
Posterior Odds |
Comparing infinite Hypotheses
To come soon.
Have another use case I’m not covering? Let me know.
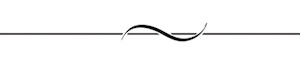
Interested in learning more?
You might also like
- How to setup duration based profiling in Sentry
- How to simulate a broken database connection for testing in Django
- Building your own Hey email Feed in Gmail
- Equivalent Salary Calculator By City